Introduction
There is a long history of the Artificial Intelligence (AI) concept. A few progressive guarantees of AI replacing human work within a few decades have existed since the 1950s. In any event, it turned out that the achievement of knowledge at human level was more confused, prompting a few "artificial intelligence winters," where AI enthusiasm faded. Artificial Intelligence is a human intelligence research and prototype where a wise specialist is a system that knows its situation and conducts activities that amplify its risk of development. In Artificial Intelligence, "Artificial" means objects that are generated or developed by individuals instead of normally occurring, and "Intelligence" is the ability to frame strategies to achieve goals by subsequently associating with a data-rich world. In essence, man-made consciousness speaks to machine knowledge and the partition of software engineering that is objective to generate it.1
Organizations are getting included and integrated with various scholastic endeavors. For a few medical science applications, such as Watson for Oncology and Watson for Genomics, IBM has developed Watson, and there are a large number of new businesses tending to any conceivable range of the image of healthcare.2
Artificial intelligence is not new, but the field has recently made rapid progress. To a limited extent, this has been empowered by advances in registering power and the tremendous volume of currently generated computerized knowledge. With expansive open and private speculation and premium, wide scopes of AI uses are currently being researched. Significant innovation organizations, including Google, Microsoft and IBM, are pouring resources into the development of AI for medicinal services and research. The quantity of AI new businesses has likewise been relentlessly expanding. AI could help organize patient courses or treatment strategies better, and furthermore furnish doctors with truly all the data they need to make a magnificent choice in medical science and drug. AI officially settled a few human resources regions and it is only the beginning to drastically transform starting from the structure of treatment strategies by strengthened boring jobs towards advancement of medical science or medication. Specialists do not need to learn just as much more material by heart as they did 50 years ago. Advanced innovation has freed restorative practitioners, medical attendants and researchers to focus on higher-level psychological undertakings and patient interest with additional mental strength. We understand that machines will not be replaced by human doctors within a reasonable time period, but AI may help doctors decide on better clinical decisions or even supplant human judgement in a certain utilitarian zones of medicinal services (e.g., radiology or neurology). The rising accessibility of healthcare information and the rapid improvement of big data analysis methods have made AI's ongoing productive applications in medicinal services conceivable. Incredible AI techniques, led by critical clinical investigations, will open up clinically relevant data covered in the enormous amount of knowledge, which can thus assist basic clinical leadership.3
The use of AI (machine learning) ML strategies, including artificial neural networks (ANNs), is another way to deal with AI. Using an ANN approach, computer commands create an artificial "neurons" decision-making system that works in ways comparable to organic sensory systems.
The primary contrast between an ML AI and an AI specialist structure is that the former is not explicitly determined by experts, but rather by a robotic adaptive upgrade process (directed or unsupervised by human specialists). By utilizing "training" datasets that match examples of information sources, (for example, side effects, therapeutic pictures, or biomedical sign) with indicated yields, (for example, medicinal determinations), the ML program can iteratively realize which system will coordinate the most patterns accurately.
In medical science healthcare, ML-fueled clinical decision support system (CDSSs) had been proposed for picture survey and translation for radiology, dermatology, and pathology, and for improving the extension and precision of biomedical sign interpretation. There are a few concerns about this ongoing history of rapid automation improvements, as AI is linked to the context of human health. Medicinal services are a sector of welfare protection. For example, AlphaGo and IBM Watson have won prominent game shows or rivalries, rather than the low-risk innovation shows methodologies, the medicinal services network will expect a higher level of receptivity and approval before AI-based devices are adopted.4
Early research
The most punctual uses of AI in the restorative field occurred during the 1970s, as stated in old history books (the term itself having been instituted by John McCarthy in 1955). Early scholars such as Alan Turing first discussed on a larger scale whether PCs could be made to function in a manner close to that of the human cerebrum (i.e., to think).(6)
Healthcare data
They should be 'trained' by knowledge generated from clinical activities, such as screening, commitment, treatment task, etc., before AI structures can be sent in healthcare applications, in order to learn comparable subjects meetings, relationships between subject highlights and premium outcomes. This clinical information often exists in the form of socioeconomics, restorative notes, electronic medical gadget accounts, physical examinations, and clinical laboratories and images, which are not yet limited.5
Business Challenge in Healthcare
For personalized and virtual consideration, the digitization of medical science knowledge and the hope gradually drawn among patients are familiarizing unusual open doors with disturbing the way in which medicinal services and treatments are transmitted. The emergence of new, rich sources of knowledge, such as social determinants, genetic and imaging information, information from therapeutic gadgets and wearables and web-based life information, overcomes medicinal services frameworks. In the meantime, companies in the life sciences are facing expanding weight from the industry and administrative offices to integrate certifiable knowledge across the pharmaceutical life cycle, from clinical preliminaries to the marketing of medication products.5
Associations in healthcare and medical sciences need to change the way they use and provide data to assuage weight on human services frameworks, increase the capacity of restorative decision-making, and achieve innovative rapid recovery therapies.6
How AI can help?
For example, ML AI, Deep AI, NLP, and gauging and enhancement will unleash the full knowledge capacity to understand a portion of our most prominent health provocation and dramatically improve the way medicinal services and treatments are communicated. 6 This fundamental progress would empower associations in human services and life sciences to:
Better healthcare results: Enable doctors to be diagnosed with increasingly accurate diagnostics and concentrate on avoidance skills.
Assure medical drug safety: Empower pharmaceutical manufacturers to quickly assess the consistency, adequacy and protection of new product expectations.
Get new treatments for faster recovery and market growth: Clinical preliminaries of Quicken using sources of certifiable knowledge.
Control costs: Improve medical clinics' clinical presentation by improving staffing assets and making a change to reduce the number of readmissions from emergency clinics.
Artificial Intelligence and Healthcare
The information dependent of artificial intelligence has huge consequences for human health. Similar to the entry and use of medicinal data, this industry is very much managed. With AI, framework consistency is simpler. In reality, as we know where calculations can be analyzed, wearable gadgets can follow essential signs and robots can be controlled remotely to perform surgeries, will tomorrow's clinicians become a threatened species in the long run? Simulated intelligence may be the area of the world's largest innovation organizations, but if AI devices are to be recognized to drive tolerant engagement, support and suggestion from clinicians is still required. The future task of medical professionals will not be to separate data from images and histology with the support of AI instruments, but to deal in the clinical environment with the data extracted by AI. Ideally, artificial intelligence will place the part of humanity' back in medicinal services by allowing clinicians to focus on the patient instead of being suffocated by voluminous data. An article, "Medical procedure, Virtual Reality, and the Future" author emphasized that AI should actually have the problems specialists in a perfect world location, not what engineers think they have. Technology that adjusts to and reliably speaks to the anatomical, utilitarian and physiological status of the patient should be updated. It is only necessary to provide information that is needed, and that too, only when necessary. Work processes should be stepped up, not reclassified.7
There seems to be a close distance to a situation where medicinal data, assembled for the purpose of consideration, is broken down using complex machine calculations to provide ongoing significant investigation. The formation of information-driven expectations promotes general well-being with customized medication and accuracy. The biggest challenge is to interpret specialized achievements to have a significant clinical effect. Organizations among clinicians and information researchers, upheld by the strength of clinical informatics, are continuing to produce positive outcomes AI requires an intensive and accurate assessment prior to incorporation into the routine clinical consideration; its potential for causing a colossal effect should not be underestimated in any case, as other problematic innovations previously.8
Applications of AI in Healthcare
Potential clinically considered AI roles include:9
Clinical care
Computer-based intelligence can potentially assist in determining sickness and is currently being tested in some UK clinics for this reason. Likewise, the use of AI to examine clinical information, research publications, and expert rules could inform treatment choices.10
Medical imaging
Therapeutic outputs have been collected and put away methodically for quite a while and are readily available for the preparation of AI systems. The cost and time associated with examining filtration could be reduced by AI, conceivably allowing more sweeps to be taken to the more likely target treatment. In identifying conditions, for instance, pneumonia, skin and breast cancer, and eye ailments, AI has shown promising results.11
Echocardiography
Tested in research, the Ultromics framework utilizes AI to investigate echocardiography filters that identify pulse examples and analyze coronary disease.
Screening for neurological conditions
AI systems are being created to analyze samples of discourse to predict insane scenes and detect and track side effects of neurological disorders, such as Parkinson's infection, for example.
Surgery
AI-constrained automated tools have been used in research to complete complex tasks in keyhole surgery, such as tying knots to close injuries.
Image based diagnosis
As of now the best field of medical AI applications is potentially computerized restorative picture analysis. Numerous restorative fortes depend on picture-based determinations, including radiology, dermatology and pathology. In what endeavors, we condense to each of these late advances in the use of AI.
AI in radiology
In the current century, the radiologist's perception assumes an inexorably more notable role of patient decision power and patient consideration. An increased incidence of chronic diseases requiring continuous imaging has led to increasingly complex sweeps, more output cuts, and much higher targets, accompanied by an extreme shortage of radiologists, an ideal formula for delayed elucidation and longer overview hold-up time.12
The critical test that perseveres for a radiologist in such circumstances is an expansion in the level of effort to produce further yields without relying on the degree of precision in their diagnosis.12
Numerous medicinal imaging modalities are used by analytical radiologists to identify and examine diseases, the most commonly used being X-ray radiography, processed tomography, magnetic resonance imaging (MRI) and positron emanation tomography. Radiologists use a series of photographs for disease screening and determination in both of these methodologies, to recognize the cause for illness and to screen the course of the patient over the duration of an illness.
Radiological practice is essentially based on recognition imaging and is entirely manageable for deep learning systems along these lines, as images routinely contain an enormous amount of data required to arrive at the correct determination. In a picture archiving and correspondence method, most radiology divisions maintain a database of verifiable images, which typically gives a large number of guides to prepare the neural systems.13
Numerous radiology uses AI, such as the identification of lung knobs using registered tomography images, the diagnosis of aspiratory tuberculosis and basic lung diseases with chest radiography and breast mass recognition using mammography scans, have achieved master-level analytical correctness with the aid of current AI techniques. These investigations used a process known as transfer learning, where settled deep neural systems are obtained prepared on a large number of characteristic, non-medicinal images, and then the connections of the neural system are tweaked by using a large number of biomedical images. Such a method can minimize the amount of test training needed to train a neural network with a large number of parameters, making it profoundly effective in characterizing clinical images where the number of images must be thousands.14
AI in dermatology
The review assumes a major role in the diagnosis of various forms of skin injury. Regular skin melanoma, for instance, has visual highlights that distinguish these sores from benign moles. Dermatologists have created general guidelines for the conclusion of skin melanoma by investigation, such as the commonly recognized ABCDE law. The standard is associated with the diagnosis of pigmented tumors, where Foundation A refers to the tumor's geometric asymmetry, B to sporadic fringes, C to shading variegation, D to a diameter equal to or greater than 6 mm, and E to the increase of the outside of the injury or progressing lesion. With the exception of the E norm, various requirements from a solitary picture of the sore may be tested. 14
Specialists have been trying for a long time to build robotized indicative structures that order images of benign and malignant lesions. As of late, in diagnosing skin malignancy, convolutionary neural systems prepared on 129, 450 clinical images achieved dermatologist-level accuracy. In an analysis of the measurement standards and the evaluations by 21 dermatologists on several photographic and dermoscopic images, the deep learning calculation outflanked the normal dermatologist. Despite the fact that the preparation period of the profound learning model may be computationally expensive, it is possible to transmit the finished analytical model on mobile phones, conceivably increasing the openness of skin lesion screening globally at the master stage. 10
The majority of AI use cases and the development of dermatology applications seem to fall into two main groups:
AI in pathology
For the diagnosis of various cancer types, histopathological assessment is the highest standard of consistency.15 The system involves treating tissue slides with a biopsy or surgical example and recoloring the slides with colors, and then translating the slides under a magnifying instrument based on visual examination by the master pathologist. Nevertheless, differences between pathologists have been reported, and the approach is not efficiently adaptable. In addition, some quantitative histopathology images that are barely observable by the human eye may anticipate the survival outcomes of disease patients in the pathology slides showing the existence of rich but already underused evidence.16 AI may be useful in the detection of prostate cancer from biopsy specimens, the identification of breast cancer metastasis in lymph nodes, and the detection of mitosis in breast cancer with the approach of profound convolution neural networks. For example, AI may promote hazard stratification of prostate and breast cancer patients in combination with a framework for imaging live-cell biomarkers. A computerized framework could reduce this shortfall, provide a fast and targeted evaluation of the histopathology slides, and enhance the nature of consideration for cancer patients, as it is assessed that there will be a net shortfall of over 5,700 full-time proportional pathologists by 2030.
In order to achieve master level symptomatic accuracy, successful applications in radiology, dermatology, and pathology influence the accessibility of enormous marked knowledge, calculation capacity, and deep learning strategies by and large. It is not clear to view these test outcomes in clinical applications, but it may probably fully alter current medical practice.16
AI in drug development
The initial stage in the growth of drugs is the discovery of novel chemical elements with organic action. From the cooperation of the compound with a specific catalyst or with an entire living being this natural movement will arise. A 'hit' is defined as the primary compound that shows motion against a given organic target. During the screening of substance libraries, PC recreation or screening of usually detached items, for example, plants, microbes and organisms, hits are regularly identified. 17
Identifying lead molecules is the second step. A lead is a chemical element that shows promising potential that can cause the advancement as a cure for a disease or another drug. In cell-based measures prescient of the diseased condition and in creature models of infection, few known hits are screened to represent the viability of the compound and its possible well-being profile. If a lead compound has been discovered, its material structure is used as a starting point for chemical modifications with the intention of finding mixes of maximum remedial benefit and negligible potential for mischief.18
Applications of AI in drug development
AI in synthesis of drug-like compounds
AI in drug repurposing
AI in selection of a population for clinical trials
AI in finding the hit or lead
AI in cardiology
In cardiology, the guarantee of AI and ML is to deliver a lot of devices to increase and extend the cardiologist's adequacy. For a few reasons, this is needed. For example, the clinical presentation of information-rich developments, entire genome sequencing and gushing cell phone biometrics would expect cardiologists to translate and operationalize data from various specific biomedical fields before long. At the same time, installing outer drug weights requires more influential practical efficacy from doctors and healthcare frameworks. Finally, patients are beginning to call for faster and more customized consideration. Doctors are thus immersed in data that needs increasingly refined elucidation, thus depending on it to function all the more productively. The solution is AI, which from research and disclosure to conclusion to treatment determination will upgrade each step of patient consideration. Clinical practice can therefore turn out to be increasingly effective, gradually supportive, gradually customized, and gradually convincing. Furthermore, knowledge about the future will not be gained exclusively through the human services system. The proliferation of portable sensors will allow doctors to screen, interpret and respond to additional surges of biomedical data collected remotely and naturally.19
Artificial intelligence has grown because it is possible to constantly boost increasingly well-known calculations for certifiable enterprises. Think of an example of a measured relapse. This model requires different solid assumptions to allow observable induction, such as the estimation of coefficients and for other calculations. (e.g. freedom of perceptions and no multicollinearity among factors). The assumptions that empower statistical inference may be random to the objective at the point where strategic relapse is used for various purposes and may inhibit the presentation of the model. Interestingly, without having the same numbers of suspicions of the fundamental details, AI calculations are normally used. Despite the fact that this approach ruins the possibility of normal measurable derivation, it produces equations that are increasingly precise for expectation and characterization for the most part. The combination of AI and ML can also benefit from cardiovascular medication. 6
Virtual Branch
Machine Learning (also known as Deep Learning) speaks to the virtual component that is addressed through numerical calculations that enhance learning through experience. There are three types of AI calculations: I unsupervised (capacity to discover designs), (ii) supervised (past model-dependent grouping and forecast algorithm), and (iii) fortification learning (utilization of arrangements of remunerations and disciplines to frame a technique for task in a particular issue space). To begin with, AI has supported is still boosting discoveries in hereditary characteristics and atomic drug, by giving AI algorithm and knowledge to the board. However the new "frameworks thinking" on human services not only focuses on the old-style relationships between patients and suppliers, but recognizes broader connections and cycles of size.20
In addition, the system for medicinal services must not be stationary, but rather should benefit from its own encounters and aim to incorporate consistent process improvements. This is a multi-agent method (MAS), where several agents interact with each other structured in a typical domain. This procedure involves producing or engaging in an association that uses AI to make noteworthy improvement.21 The improvement of hazardously complex environments for treating interminable mental infections is a case of such a treatment of medicine. Instead of focusing on intake of well-being (in general well-being structures) or cost recovery (in the board well-being of association) the MAS method proposes to capture the components of individual patients, including their reactions to drugs and their behavioral responses within a broader biological system in society. This global consideration of creativity in teamwork enables process of mapping, promotes control, and increases support for improvements to the system with a demonstrated increase due to medication, cost reduction, and increasingly strong mediation. Its use has helped supervisors of well-being systems to break down the elements of system execution through changes in social, clinical and criminal equity components.22
Electronic medicinal documents are integrated into the interactive uses of AI where explicit calculations are used to identify subjects with a family ancestry of a genetic disorder or an increased risk of a ceaseless disease. Computer-based intelligence is used to enhance authoritative execution by enabling individuals to increasingly identify, share and apply their aggregate knowledge to make' ideal choices.' As a result, the executives are essential in achieving the ideal quality of the electronic medicine records and social insurance process. Data should be captured in a computerized configuration available as individual information from current patient record keeping of variable quality, as well as in amassed structures for epidemiological research and arrangement. In order to achieve the desired viability and limit costs, significant efforts from the academic world and the data innovation industry are required.
The current status of therapeutic records is generally for the health framework and for learning security as unknowable storehouses of lost data. To speed up the execution of electronic wellness records, research facilities and centers need to team up. Information should be gradually captured, and organizations should advance their transition into understandable procedures.23 New logical and clinical results can be exchanged by open-source, and complete data must be shown by doctors and researchers for open access and thereby made usable for data consideration purposes. Combination and compatibility, including moral, legal and calculated issues, are monumental, particularly with the expected "omics-based" knowledge expansion.24 It is important to make clear the reorganization, understandability and clinical usefulness of knowledge sets, and each result must be discussed for its clinical relevance. 5
Physical Branch
The second type of use of AI in drug incorporates physical articles, restorative gadgets and progressively complex robots partaking in the conveyance of consideration. The use of robots as aids is perhaps the most motivating methodology; for example, robots buddy with subjective decrease or restricted portability for the maturing population. As associate experts or even as solo entertainers, robots are used in medical procedures.25 The ability to talk to and view medically introverted young people is one of the most amazing examples of the usefulness of robots. Important moral considerations should be resolved here, and in several different cases that may benefit from automated mediation, until it is feasible to regularly use AI robots in the current clinical situation. Aside from moral concerns, the need for systemic, close evaluation of the effect of electronic systems on well-being markers and proportions of changes in mental and physical condition, responses, and outcomes is a notable test in this new aspect of therapeutic consideration.26
In an assortment of prescription courses, artificial intelligence may be used. Four models are given here:18, 27
Clinical information analysis
Approximately 80 percent of information in human services is unstructured and AI can peruse and understand unstructured information. The ability of artificial intelligence to process common language allows it to peruse clinical content from any source and distinguish therapeutic and social ideas from order and code.
Knowledge for patient information
AI can discern the problems found in the actual clinical records of patients, both in the organized and unstructured material. It explains the historical context of their consideration of these problems and may provide a medical record with a psychological synopsis.
Patient similarity
A proportion of clinical comparability can be differentiated between patients by artificial intelligence. As compared to static patient partners, this helps scientists to make complex patient accomplices. It also allows an appreciation of which treatment approach fits best for a particular group of patients.
Therapeutic section of knowledge
Experts can uncover material in unstructured medical writing with AI innovations to endorse hypotheses, helping to expose new experiences. Artificial intelligence, for example, Medline, will peruse a complete arrangement of restorative writing and identify the documents that are semantically associated with some combination of therapeutic ideas.
AI in neurosciences
Brain tumor removal has been accepted as a perfect robotic surgery technique. It involves a view of the planet by means of an automated system and a subsequent adaptation of its behavior to evolving natural parameters. Surgery knot tying is also a test for sending AI.28 Among people, the shape and precise area of engine cortical regions varies. For the organization of neurosurgical methodology, definite learning of these areas is necessary. To inspire engine-evoked potential reactions reported for individual muscles, Robot-helped picture directed transcranial magnetic stimulation (Ri-TMS) was used to recreate useful engine maps of the critical engine cortex.26 For a neurosurgical resident, it is increasingly becoming difficult to learn" about a patient in the OT. The need for functional neurosurgical recreation is of great significance. With a section of a virtual three-dimensional (3D) ventricular catheter through the brain parenchyma into the ventricle, a PC-based, computer-generated experience stage provides imitated obstruction and unwinding. The advancement of AI and haptic science and innovation (perceiving objects through touch) enhances the learning of clinical skills and methods.27
AI in neurosurgical OT:
Currently, it is recognized that cerebral tumors cause a significant redesign of the functional system. Useful enrollment has been helped by simulated intelligence showing greater optimum percentage than anatomical enlistment. Functional limitation of enacted yet uprooted districts is essential, particularly in situations where hemodynamic tumor-induced changes make direct containment problematic.29 Testing is to coordinate functional brain districts crosswise over individuals, to a large extent due to fluctuations in their area and degree. Pathology expansion can trigger considerable rearrangement of useful frameworks. This issue is addressed by advances in the neural data processing framework using AI.30
AI in cancer
Cancer growth offers a unique setup for restorative choices not only because of its varied structures with infection progression, but also because of the need to consider the individual condition of patients, their ability to receive treatment, and their reactions to treatment. Most commonly, radiographic assessment of disease relies on visual evaluations, the understandings of which could be broadened by cutting-edge computational investigations. In particular, Artificial Intelligence (AI) promises to take incredible steps in the subjective translation by master clinicians of malignant growth imaging, including volumetric outline of tumors after some time, extrapolation of the tumor genotype and natural course from its radiographic phenotype, clinical outcome prediction, and assessment of the impact of infection and treatment on contiguous organs.27
In the underlying translation of images, artificial intelligence will be in robotize forms and pass the clinical process of radiographic exploration, the executive decisions on whether to monitor a mediation, and the subsequent interpretation to a worldview that has yet to be conceived. Here as linked to medicinal imaging of disease, the creators survey the current domain of AI and show propels in 4 types of tumors (lung, cerebrum, breast and prostate) to reflect how normal clinical problems are being treated. Research evaluating of AI applications in oncology have not been overwhelmingly accepted for reproducibility and generalizability, the outcomes are increasingly organized in driving AI innovation to clinical use and impacting future cancer care headings.29
As a self-sustaining and flexible procedure that interacts strongly with its microenvironment, cancer continues to destroy patients, analysts, and clinicians, despite tremendous progress in understanding its natural foundations. With this multidimensional nature, problems arise at each stage of the disease, including a solid early location; accurate distinction of pre-neoplastic and neoplastic injuries; assurance of infiltrative tumor edges during careful treatment; after some time of tumor growth and possible safety from medicines; and expectation of tumor intensity, metastasis examination are done. In the range of malignancy position, treatment, and observation, mechanical advances in therapeutic imaging and insignificantly invasive biomarkers ensure that certain problems are addressed. Nevertheless the understanding of the enormous volume of data produced by these developments reveals a blast of new potential difficulties. 11
As we examine the disease itself, we are becoming familiar with the intensity of resources that are now available to us, which could be used in exceptional ways. It should be recognized by non-neoplastic enhancers at the stage where a neoplastic sore is first detected and ordered to advance the form and power of treatment based on its intended clinical course and organic forcefulness. Accidental detection of injuries within the body has been filled with uncertain clinical meaning by the far-reaching accessibility of processed tomography (CT) and attractive magnetic resonance imaging (MRI), which at that point begins a path of interpretation, further testing, or scientific mediation. With treatment, which includes cyto-reduction by medical procedure, elicitation through radiation of immediate and circuitous components of tumor destruction, and pharmacotherapy, cancers may respond to forced, growing, and repeating stressors. Qualification must be made between neoplasm or tissue reaction to injury, with the radiographic appearance of a sore that increases in size after treatment. Neoplastic sores have repeatedly been shown to contain new sub-atomic deviations specific to the critical tumor that could offer protection from restorative or radiation therapy. This is compounded by the inherent intra-tumor heterogeneity of tumors at the time of initial discovery, which is increasingly seen in regular clinical obsessive testing and profiling by study yet hard to identify. The interest in non-invasive imaging has never been more evident, as the most commonly accepted technique for following reactions to treatment and proposing basic data on tumors themselves. 19
Conventional radiographic tumor imaging evaluation depends to a large extent on subjective highlights, such as tumor thickness, upgrading example, intra-tumor cell and a cellular piece (counting for blood proximity, corruption, and mineralization), tumor edge normality, anatomical tissue relationship, and impact on these structures. In 1--, 2--, 3-dimensional investigations, size-based and shape-based proportions of the tumor can be measured. All aspects known to be called 'semantic' highlights are these subjective phenotypic representations. A rapidly developing area called radiomics in the study enables computerized deciphering of radiographic images into quantitative highlights, including form, scale, and textural pattern descriptors. Latest developments in man-made brainpower (AI) techniques have taken extraordinary measures in determining radiographic examples in medical imaging data as a result. Profound learning, a subset of AI, is an especially encouraging technique that naturally highlights representations from test images and has emerged in task-specific applications to organize and even exceed human execution. Despite the need for enormous knowledge indexes for training, deep learning has demonstrated relative strength in ground truth labels, among others, against clamor.11 AI has computerized capabilities which give the ability to improve clinicians' subjective skills, including correct volumetric tumor size outline after some time, parallel follow-up of multiple accidents, analysis of intra-tumor phenotypic complexities to genotype recommendations, and outcome expectation by cross-referencing singular tumors to databases of conceivably limitless similar cases. In addition, deep learning methodologies ensure more influential cross-cutting generalizability over ailments and imaging modalities, intensity to noise, and error reduction, eventually causing prior intercessions and vital enhancements in determination and clinical consideration. Despite the fact that these tests remain to a large extent in the preclinical research space, the development of such programmed radiographic 'radiomics' biomarkers can present clinically relevant changes in tumors and after some time, lead to a change in outlook in the segregation of malignant growth. 8
At the beginning of this energizing mechanical transition, in 4 basic malignant growth types: lung, cerebrum, bosom, and prostate disease, we review the existing evidence and future headings for AI approaches linked to restorative imaging. In the identification and treatment of malignancy, we represent clinical challenges and constraints, how existing strategies are attempting to resolve these, and how AI can influence future bearings.31
AI applications in cancer imaging
The desire to enhance the adequacy and quality of clinical consideration continues to drive different technologies, including AI, into work. The enhancement and streamlining of clinical work processes has become progressively fundamental with the constantly increasing interest of medicinal services administrations and the tremendous volumes of knowledge provided every day from parallel streams. Artificial intelligence exceeds standards of perceiving complex image examples and thereby provides the ability to transform the interpretation of images from a fully subjective and emotional undertaking to a quantifiable and easily reproducible one. Similarly, AI will calculate data from images that people do not interpret and support basic clinical leadership accordingly. In addition, artificial intelligence will allow the aggregation of various streams of knowledge into incredible organized demonstration structures that spread over radiographic images, genomics, pathology, electronic records of well-being, and interpersonal organizations.32
AI reveals incredible usefulness in performing 3 primary clinical tasks in cancer imaging: tumor identification, depiction, and observation. Discovery refers to the restriction of objects of radiograph enthusiasm, all things considered computer-aided recognition (CADe). Identification devices based on AI can be used to decrease observational oversights and fill in as an underlying screen against omission errors. Areas with suspicious imaging qualities are presented and shown to the reader within a pattern-recognition environment. CADe has been used as an assistant associate to detect missed cancer in low-dose CT screening, identify mental metastases in MRIs to enhance the translation time of radiology while retaining high sensitivity to discovery, find micro calcification bunches as a predictor of early bosom carcinoma in screening mammography, and all the more, radiologist affectability has been enhanced to distinguish between early chest carcinoma.23
Figure 6
Applications for computerized reasoning in medicalimaging as applied to common cancers to refer to 3 general classifications ofimage-based clinical assignments in oncology, AI reasoning methods can bedefined: 1) discovery of variations from the norm; 2) depiction of a presumed sore by characterizing its shape or length, histopathologic examination, disease process, or atomic profile; and 3) assurance of anticipation or reaction to treatment after some time during observation.
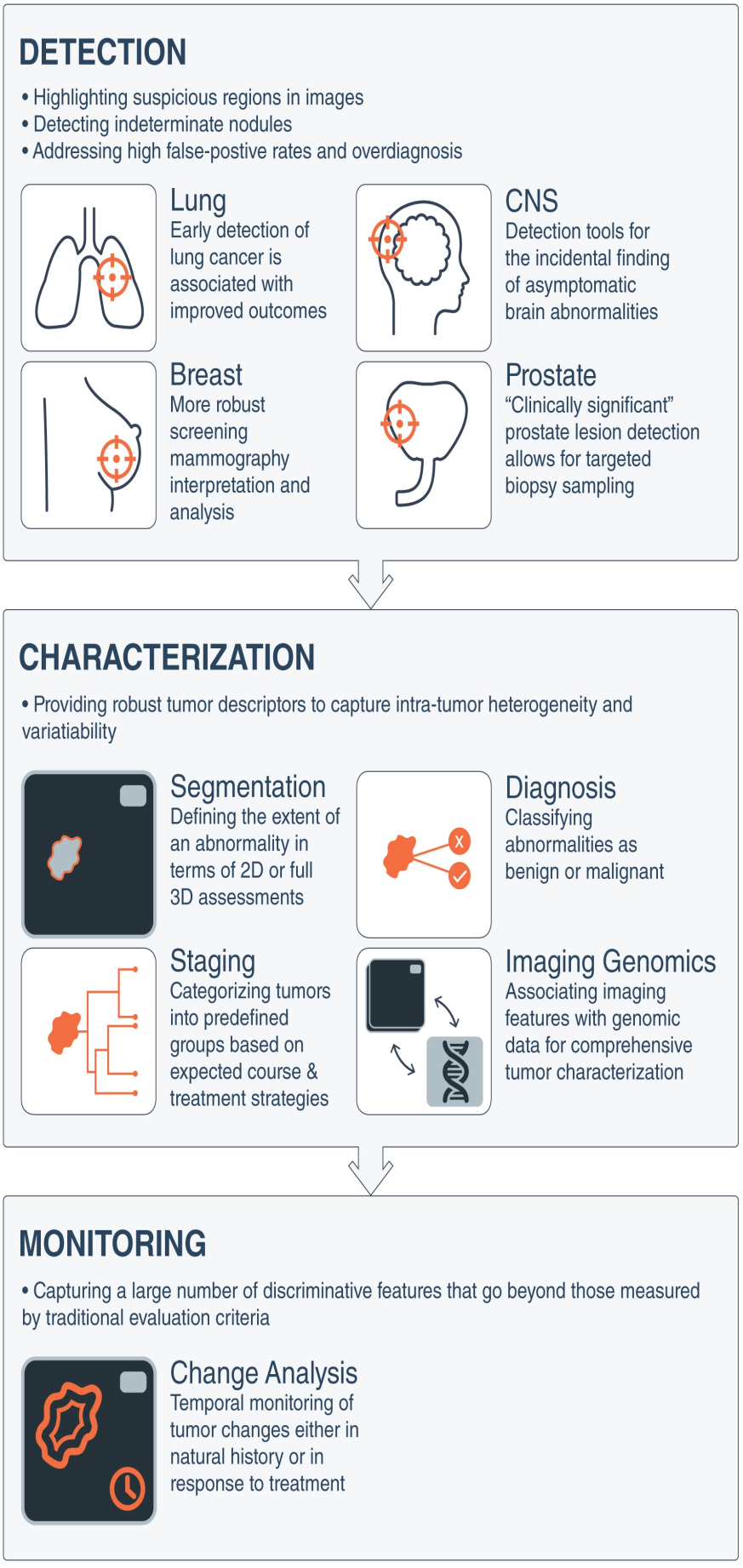
Individual health virtual assistant
The overwhelming majority are approaching a mobile phone in the present age. They're probably going to have their mobile phones with their menial helpers. Propelled AI equations, such as Cortana, Google Assistant, and Siri, influence colleagues. They can offer tremendous benefits to consumers at the moment when combined with healthcare applications. As an individual well-being associate, medicinal services applications will go around. They will also be used to provide drug warnings, and it will also be conceivable for human-like cooperation. Artificial intelligence will also help to assist patients when the clinical faculty is not available as an individual partner. 25
Artificial intelligence improves the quality of sleep
It has been shown that a pleasant night's rest is important for better physical and psychological well-being. Throughout the day, individuals who get sound rest during the night are more happy, more beneficial, and increasingly profitable. There are great deals on the market for viable rest facilities that help you rest better at night. AI innovation is constantly trying to improve the general nature of rest, from AI controlled savvy bedding to infant screens, rest applications. Two bedtime songs were made by a medical services organization named AXA PPP, one by AI and the other by individuals. The AI framework could figure out mood and concordance with profound learning to bring about another organization. This piece was then transformed with the help of a human into a melody, and it can allow you to rest better.14
Medicinal imaging analysis
Radiology is another significant field in medicinal services that utilises AI. With analytical procedures, AI frameworks can help. It can look at therapeutic images such as X-beams, CT reviews, MRIs, and so on and can criticise what a human eye can skip.
Therapeutic imaging research, along these lines, turns out to be significantly more accurate and viable. The odds of blunders are decreased. IBM Watson is a model for a livelihood. It can provide clinicians with proof-based treatment options for cancer patients in the field of oncology, depending on the preparation. 3, 31
Precision medicine
Genomics is the part of atomic science that manages genome structure, growth, ability, and mapping. It searches for the connections from the data obtained from the DNA to the disease.33
It is conceivable to detect cancer and some vascular infections at the moment when combined with AI at an all-around starting period. It can also predict the medical issues that patients may face, depending on their characteristics. AI also assists in the machining of medicinal parts in this way, minimizing the chances of operation malfunction.24
Healthcare bots
Innovation in artificial intelligence is also making inroads in the customer service space. The world is going to see healthcare machines very soon, undoubtedly. Patients can almost probably interact through a talk window or by phone with these AI bots on the web. Social insurance bots can be used to arrange meetings with the provider of medicinal services for the patient. Bots may also support patients with their medication. By providing 24 x 7 assistance, they will likewise boost customer service.34
These are a fraction of the awesome things that AI can do. It's not limited to that in any event. If innovation extends the boundaries of human services, it will be feasible to provide better answers for spare time, resources, and professionalism.35